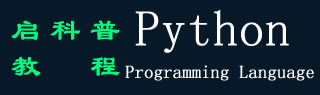
- Python 菜鸟教程
- Python 教程
- Python - 概述
- Python - 历史
- Python - 特性
- Python 与 C++
- Python - Hello World 程序
- Python - 应用领域
- Python 解释器及其模式
- Python - 环境设置
- Python - 虚拟环境
- Python - 基本语法
- Python - 变量
- Python - 数据类型
- Python - 类型转换
- Python - Unicode 系统
- Python - 文字
- Python - 运算符
- Python - 算术运算符
- Python - 比较运算符
- Python - 赋值运算符
- Python - 逻辑运算符
- Python - 按位运算符
- Python - 成员资格运算符
- Python - 身份运算符
- Python - 运算符优先级
- Python - 注释
- Python - 用户输入
- Python - 数字
- Python - 布尔值
- Python 控制语句
- Python - 控制流
- Python - 决策
- Python - if 语句
- Python - if-else 语句
- Python - 嵌套 if 语句
- Python - Match-Case 语句
- Python - 循环
- Python - For 循环
- Python for-else 循环
- Python - While 循环
- Python - break 语句
- Python - Continue 语句
- Python - pass 语句
- Python - 嵌套循环
- Python 函数和模块
- Python - 函数
- Python - 默认参数
- Python - 关键字参数
- Python - 仅关键字参数
- Python - 位置参数
- Python - 仅位置参数
- Python - 任意或可变长度参数
- Python - 变量范围
- Python - 函数注释
- Python - 模块
- Python - 内置函数
- Python 字符串
- Python - 字符串
- Python - 切片字符串
- Python - 修改字符串
- Python - 字符串连接
- Python - 字符串格式化
- Python - 转义字符
- Python - 字符串方法
- Python - 字符串练习
- Python 列表
- Python - 列表
- Python - 访问列表项
- Python - 更改列表项
- Python - 添加列表项
- Python - 删除列表项
- Python - 循环列表
- Python - 列表推导式
- Python - 排序列表
- Python - 复制列表
- Python - 联接列表
- Python - 列表方法
- Python - 列表练习
- Python 元组
- Python - 元组(Tuple )
- Python - 访问元组项
- Python - 更新元组
- Python - 解压缩元组项
- Python - 循环元组
- Python - 联接元组
- Python - 元组方法
- Python - 元组练习
- Python 集
- Python - 集(sets)
- Python - 访问 Set Items
- Python - 添加 Set Items
- Python - 删除 Set Items
- Python - 循环 Set Items
- Python - 联接 Sets
- Python - 复制 Set
- Python - Set 运算符
- Python - Set 方法
- Python - Set 的练习
- Python 字典
- Python - 字典
- Python - 访问字典项
- Python - 更改字典项
- Python - 添加字典项
- Python - 删除字典项
- Python - 字典视图对象
- Python - 循环字典
- Python - 复制字典
- Python - 嵌套字典
- Python - 字典方法
- Python - 字典练习
- Python 数组
- Python - 数组
- Python - 访问数组项
- Python - 添加数组项
- Python - 删除数组项
- Python - 循环数组
- Python - 复制数组
- Python - 反向数组
- Python - 对数组进行排序
- Python - 连接数组
- Python - 数组方法
- Python - 数组练习
- Python 文件处理
- Python - 文件处理
- Python - 写入文件
- Python - 读取文件
- Python - 重命名和删除文件
- Python - 目录
- Python - 文件方法
- Python OS 文件/目录方法
- Python - os.path 方法
- 面向对象编程
- Python - OOP 概念
- Python - 类和对象
- Python - 类属性
- Python - 类方法
- Python - 静态方法
- Python - 构造函数
- Python - 访问修饰符
- Python - 继承
- Python - 多态性
- Python - 方法覆盖
- Python - 方法重载
- Python - 动态绑定
- Python - 动态类型
- Python - 抽象
- Python - 封装
- Python - 接口
- Python - 软件包
- Python - 内部类
- Python - 匿名类和对象
- Python - 单例类
- Python - 包装类
- Python - 枚举
- Python - 反射
- Python 错误和异常
- Python - 语法错误
- Python - 异常处理
- Python - try-except 块
- Python - try-finally 块
- Python - 引发异常
- Python - 异常链接
- Python - 嵌套 try 块
- Python - 用户定义的异常
- Python - 日志记录
- Python - 断言
- Python - 内置异常
- Python 多线程
- Python - 多线程
- Python - 线程生命周期
- Python - 创建线程
- Python - 启动线程
- Python - 联接线程
- Python - 命名线程
- Python - 线程调度
- Python - 线程池
- Python - 主线程
- Python - 线程优先级
- Python - 守护程序线程
- Python - 同步线程
- Python 同步
- Python - 线程间通信
- Python - 线程死锁
- Python - 中断线程
- Python 网络
- Python - 网络编程
- Python - 套接字编程
- Python - URL 处理
- Python - 泛型
- Python 杂项
- Python - 日期和时间
- Python - math 模块
- Python - 迭代器
- Python - 生成器
- Python - 闭包(closures)
- Python - 装饰器( Decorators)
- Python - 递归
- Python - 正则表达式
- Python - PIP
- Python - 数据库访问
- Python - 弱引用
- Python - 序列化
- Python - 模板
- Python - 输出格式
- Python - 性能测量
- Python - 数据压缩
- Python - CGI 编程
- Python - XML 处理
- Python - GUI 编程
- Python - 命令行参数
- Python - 文档字符串
- Python - JSON
- Python - 发送电子邮件
- Python - 更多扩展
- Python - 工具/实用程序
- Python - 图形用户界面
- Python 高级概念
- Python - 抽象基类
- Python - 自定义异常
- Python - 高阶函数
- Python - 对象内部
- Python - 内存管理
- Python - 元类
- Python - 使用 Metaclasses 进行元编程
- Python - 模拟和存根
- Python - 猴子修补
- Python - 信号处理
- Python - 类型提示
- Python - 自动化教程
- Python - 人性化软件包
- Python - 上下文管理器
- Python - 协程
- Python - 描述符
- Python - 诊断和修复内存泄漏
- Python - 不可变数据结构
Python random.weibullvariate() 方法
Python 中的 random.weibullvariate() 方法生成遵循 Weibull 分布的随机数。Weibull 分布是连续概率分布,取决于两个正参数 alpha (α) 和 beta (β) 的值,这两个参数都必须大于 0。参数 alpha (α) 定义分布的尺度。其中,参数 beta (β) 定义分布的形状。
注意 − 这个函数不能直接访问,所以我们需要导入 random 模块,然后我们需要使用 random 静态对象调用这个函数。
语法
以下是 weibullvariate() 方法的语法 -
random.weibullvariate(alpha, beta)
参数
Python random.weibullvariate() 方法接受以下参数 -
- alpha:这是 Weibull 分布的尺度参数,它必须大于 0。
- beta:这是 Weibull 分布的形状参数,它也必须大于 0。
返回值
此 random.weibullvariate() 方法返回一个遵循 Weibull 分布的随机数。
示例 1
让我们看一个使用 random.weibullvariate() 方法从尺度参数为 2 和形状参数为 3 的 Weibull 分布中生成随机数的基本示例。
import random
# Parameters for the Weibull distribution
alpha = 2
beta = 3
# Generate a Weibull-distributed random number
random_number = random.weibullvariate(alpha, beta)
print("Generated random number from Weibull distribution:", random_number)
以下是输出 -
Generated random number from Weibull distribution: 1.9258753905992894
注意:由于程序的随机性,每次运行程序时生成的 Output 都会有所不同。
示例 2
下面是一个示例,它使用 random.weibullvariate() 方法生成并显示一个直方图,显示 scale 参数为 1 且形状参数为 0.5 的 Weibull 分布式数据的频率分布。
import random
import numpy as np
import matplotlib.pyplot as plt
# Parameters for the Weibull distribution
alpha = 1
beta = 0.5
# Generate Weibull data and convert to integers
data = [random.weibullvariate(alpha, beta) for _ in range(10000)]
plt.figure(figsize=(7, 4))
# Create a histogram of the data
plt.hist(data, bins=100, density=True, alpha=0.6, label=f'($\alpha={alpha}$), beta={beta})')
plt.title('Histogram of Weibull Distributed Data')
plt.show()
在执行上述代码时,您将获得如下所示的类似输出 -
示例 3
下面是另一个示例,它使用 random.weibullvariate() 方法生成并显示一个直方图,该直方图显示来自 Weibull 分布的数据分布。
import random
import matplotlib.pyplot as plt
# Define a function to generate and plot data for a given alpha and beta
def plot_weibullvariateian(alpha, beta, label, color):
# Generate Weibull-distributed data
data = [random.weibullvariate(alpha, beta) for _ in range(10000)]
# Plot histogram of the generated data
plt.hist(data, bins=100, density=True, alpha=0.6, color=color, label=f'(alpha={alpha}, beta={beta})')
fig = plt.figure(figsize=(7, 4))
# Plotting for each set of parameters
plot_weibullvariateian(1, 0.5, '1, 0.5', 'blue')
plot_weibullvariateian(1, 1, '1, 1', 'red')
plot_weibullvariateian(1, 1.5, '1, 1.5', 'yellow')
plot_weibullvariateian(1, 5, '1, 5', 'green')
# Adding labels and title
plt.title('Weibull Distributions')
plt.legend()
plt.ylim(0, 2)
plt.xlim(0, 10)
# Show plot
plt.show()
上述代码的输出如下 -
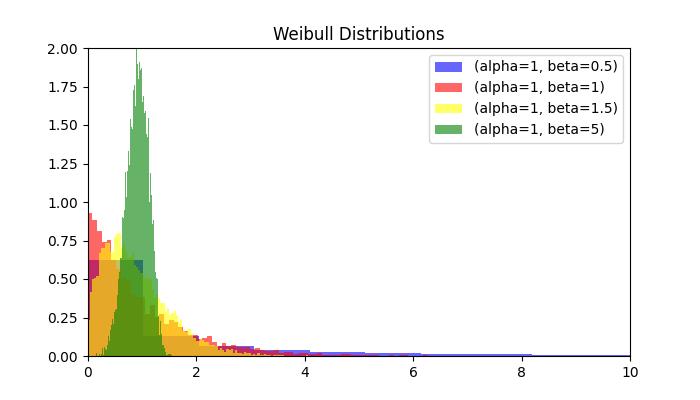